Published Research
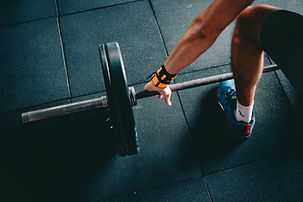
Systematic review of automatic assessment systems for resistance-training movement performance: A data science perspective
Lead Author
Traditional methods for assessing resistance training (RT) movements rely on visual checks by trainers or self-assessments, but these can be subjective and limited. New technologies using portable devices and machine learning (ML) can provide more objective assessments. This review looks at systems that use these technologies to assess RT movements for improving physical performance or rehabilitation. After reviewing 363 studies, 13 were included in the analysis. These studies showed that systems can classify movements with 70%-90% accuracy, but many of the ML models had methodological errors, and they didn’t always perform well in real-world settings. The review offers recommendations for improving future models by stressing that they should focus on providing more utility, insight, and reliability in real-world settings.

The development of an automated assessment system for resistance training movement
Lead Author
Machine learning (ML) models can automatically analyse resistance training (RT) movements like squats and deadlifts. However, previous models often lack clarity, utility, and don’t always work well in real-life situations. This study analysed more real-world-centric methodology including data collection, data processing, and modelling techniques to improve these systems. Data from 61 participants performing squats and deadlifts was collected with accessible motion capture cameras. The study found that the ML models could accurately detect certain movement errors in the squat and deadlift with accuracy over 70%, but others were less accurate (55%-60%). Results from this study suggest the proposed methodology can improve upon previous systems both from a performance and utility perspective. Future systems can benefit greatly from adopting similar philosophies in their own systems.
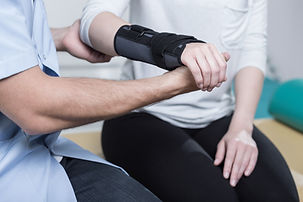
Systematic review of automatic post-stroke gait classification systems
Co-Author
After a stroke, assessing gait is crucial for guiding rehabilitation, but traditional observational methods are often unreliable and inaccurate. Data-driven systems that automatically classify gait patterns could improve accuracy, yet the development and clinical usefulness of these systems have not been thoroughly reviewed. This systematic review examines 21 studies from PubMed, IEEE Xplore, and Scopus, published between 2015 and 2022, to evaluate the methodology, performance, and clinical relevance of automatic gait classification systems. Most systems reported classification accuracy between 80% and 100%, but many had errors in machine learning (ML) model development. Additionally, many systems failed to address important factors like clinical utility, interpretability, and generalisability. The review offers recommendations for improving future gait classification systems, emphasizing the need for clinically significant models and standardised ML development practices to better support clinicians and therapists.
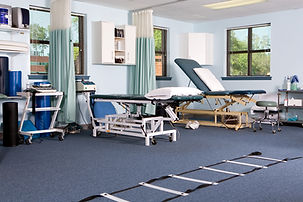
The validity of a dual Azure Kinect-based motion capture system for gait analysis: a preliminary study
Co-Author
The Microsoft Kinect can track human motion in various tasks, and the newer Azure Kinect offers improved image sensing technology. However, its validity for gait analysis is still unknown. This study developed a dual Azure Kinect motion capture system and compared its joint angle measurements with those from the Vicon system in five healthy adults. The accuracy was evaluated using the coefficient of multiple correlations (CMC) and root mean square errors (RMSE). The dual Azure Kinect system showed good accuracy for knee angles (CMC=0.87±0.06, RMSE=11.9°±3.4°) and moderate accuracy for hip sagittal angles (CMC=0.60±0.34, RMSE=15.1°±6.5°). However, it showed poor validity for hip frontal, transversal, and ankle angles. Overall, the dual Azure Kinect system showed better validity than the Kinect V2, though accuracy varied by joint. Future research should include more participants, patient populations, and sensor comparisons in a standardized setup to confirm these findings and improve the system's depth image analysis.